
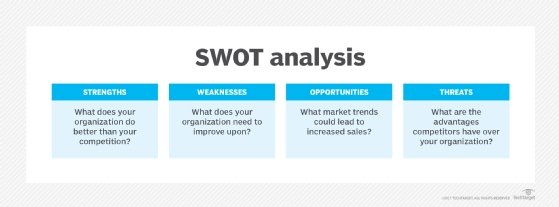
When Tableau Catalog is enabled, content authors can Search for Data by selecting from Data Sources, Databases and Files, or Tables to see if it exists in Tableau Server and Tableau Cloud and minimize duplication of data sources. For more information, see Data Management. With increased data discovery capabilities, Tableau Catalog indexes all content, including workbooks, data sources, and flows to allow authors to search for fields, columns, databases, and tables in workbooks and published data sources.
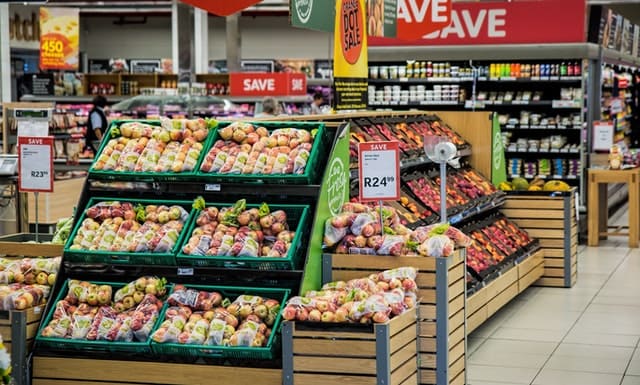
For more information, see Best Practices for Published Data Sources, Edit Views on the Web, and Optimize Data for Ask Data. Further, Published Data Sources allow Tableau Creator- and Explorer-licensed users to have access to secure, trusted data in Tableau for web authoring and Ask Data. With Tableau Data Server, which is a built-in component of the Tableau platform, you can share and reuse data models, secure how your users access data, and manage and consolidate extracts with Published Data Sources. The data source management processes you define will govern this decision. When publishing a workbook to Tableau Server or Tableau Cloud, the author will have a choice to publish the data source or leave it embedded in the workbook. For more information, see Metadata Management later in this section and The Tableau Data Model. Using relationships in well-modeled schemas can reduce both the time to create a data model as well as the number of data sources to answer business questions. Relationships are more flexible than database joins and can support additional use-cases as they arise, reducing the need to build new data models to answer new questions. Multiple levels of detail are supported in a single data source, so fewer data sources are needed to represent the same data. With multi-table support, Tableau data sources can now directly represent common enterprise data models such as star and snowflake schemas, as well as more complex, multi-fact models. They can now build multi-table data sources with flexible, LOD-aware relationships between tables, without having to specify join types in anticipation of what questions can be asked of the data. With support for a new multi-table logical layer and relationships in Tableau 2020.2, users aren’t limited to using data from a single, flat, denormalized table in a Tableau Data Source.
#Definition of analytical sandvox Offline#
In-memory extracts should be used if your database or network is too slow for interactive queries, to take load off transactional databases, or when offline data access is required. Live queries are appropriate if you have invested in a fast database, need up-to-the-minute data, or use Initial SQL. In both live and extract use cases, users may connect to your existing data warehouse tables, views, and stored procedures to leverage those with no additional work. Switching between the two is as easy as selecting the right option for your use case. Tableau’s hybrid data architecture provides two modes for interacting with data, using a live query or an in-memory extract. Without these processes, there will be a proliferation of duplicate data sources, which will cause confusion among users, increase likelihood of errors, and consume system resources. In a self-service environment, content authors and data stewards have the ability to connect to various data sources, build and publish data sources, workbooks, and other content. Tableau connects to your enterprise data platforms and leverages the governance you already have applied to those systems. Data Source Managementĭata source management includes processes related to selection and distribution of data within your organization. It creates accountability and enables, rather than restricts, access to secure and trusted content and for users of all skill levels. The purpose of data governance in the Modern Analytics Workflow is to ensure that the right data is available to the right people in the organization, at the time they need it. To define your organization’s Tableau Governance Models, you should work through the areas of data and content governance that are outlined in the diagram below using the Tableau Blueprint Planner. Just as important as defining these is having everyone in the workflow understand and comply so that users will have trust and confidence in the analytics they’ll use to make data-driven decisions. You must define standards, processes, and policies to securely manage data and content through the Modern Analytics Workflow. Governance in Tableau is a critical step to driving usage and adoption of analytics while maintaining security and integrity of the data.
